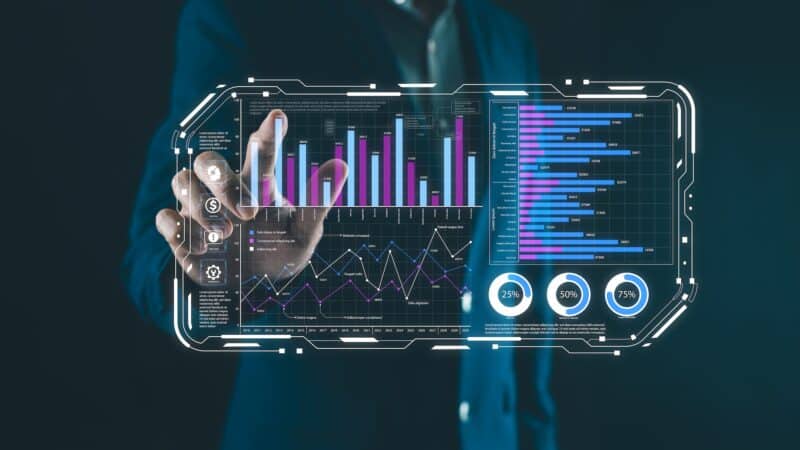
Marketers are under pressure to prove performance, but the systems they rely on are built on shaky ground. Attribution models are built on incomplete data. MMMs are often outdated and biased by design. Dashboards are full of metrics, but few deliver real insight.
The challenge runs deeper than tools or data. Measurement is held back by fragmented systems, inconsistent standards and a lack of shared accountability across the ecosystem. Until those fundamentals are addressed, even the most sophisticated solutions will fall short.
Why measurement still falls apart
Each platform handles campaign setup, activation and measurement differently, making even basic comparisons difficult. Naming conventions, attribution logic and event definitions vary so widely that even basic comparisons become unreliable.
A video platform might count a 3-second play as a “view,” while a social platform only counts full completions, yet both are labeled the same. Attribution struggles to connect the dots. MMMs slow to a crawl. And marketers spend more time cleaning data than acting on it.
The platforms marketers rely on most often provide detailed insights within their own ecosystems, but those insights don’t always translate across channels. While this can support platform-specific optimization, it makes unified measurement and cross-channel analysis more difficult.
Without shared logic and language, true measurement won’t scale.
Dig deeper: The smarter approach to marketing measurement
Structured data is the key
Marketers don’t need more data. They need structured, consistent data that ties campaign activity to outcomes — cleanly, accurately and across channels.
It starts with metadata. Not just what ran, but where it ran, who it reached and which variation was used. This context is what makes it possible to answer the real questions:
- What content environments drove results?
- Which audiences shifted behavior?
- What messages and variations worked best?
- Which formats delivered efficiency?
Too often, that context is missing or buried in manual processes. Data teams spend hours normalizing exports, decoding inconsistent taxonomies and stitching together fragmented views. That’s not analysis. It’s cleanup.
Structured data is the foundation for actionable insight. Without it, even the most advanced measurement models are just educated guesses.
Setup defines what you can measure
Even the best models fail if the campaign execution isn’t structured. How campaigns are set up (e.g., how tracking is applied, how variations are named) determines what can actually be analyzed.
Take audio. Many marketers still send static files to streaming platforms with minimal tracking. There’s no way to know whether an ad played to completion, was skipped or muted — or how different creatives performed across placements.
Better methods exist. Ads can be delivered through formats that support standardized metadata and track performance signals in real time. But platforms need to make these methods easier to adopt. Marketers and publishers need to prioritize them.
Execution isn’t just operations. It’s part of the measurement strategy.
Dig deeper: How AI and ML bridge the attribution disconnect across marketing channels
Standards aren’t the problem, adoption is
The IAB Tech Lab has built the infrastructure: shared taxonomies, transparency protocols and tools for structured, interoperable data. The IAB and MRC have created guidelines for validated, cross-platform measurement.
But too few platforms are implementing them, even fewer are independently accredited and too few marketers are pushing for them in the first place.
Platforms should offer capabilities that support structured measurement:
- Standardized fields.
- Exportable views.
- Flexible attribution logic.
- Model-ready formatting.
Marketers should be able to explore latency, compare attribution windows and audit how results are calculated, not just take the number they’re given.
Publishers need to update their systems, ad operations protocols and delivery requirements to support structured measurement by design, not as an add-on. But that shift won’t happen without pressure.
Marketers — whether on the brand side or within agencies — must start collectively pushing for these capabilities. Structure can’t be an afterthought. It needs to be embedded from the beginning in:
- Media plans.
- Tagging strategies.
- Reporting requirements.
- The overall measurement framework.
Can measurement be solved?
That depends — not on new tools or models, but on the willingness to change.
- Platforms must move beyond their own ecosystems and commit to shared standards.
- Marketers and publishers, in turn, need to demand greater transparency, interoperability, and structure.
Improving data quality is just the beginning. Measurement challenges also stem from fragmented models, complex channels and organizational silos. While better inputs won’t solve everything, they lay the foundation for meaningful progress across the board.
The technology already exists. The frameworks are in place. What’s holding us back isn’t capability — it’s mindset. Measurement won’t be fixed by another AI model. It will be fixed by prioritizing structure and aligning on consistent practices.
AI can’t fix fragmentation. Even the most advanced tools are only as good as the data they’re fed. Without clean, structured inputs, they’ll only deepen existing blind spots.
The real barrier isn’t complexity but the resistance to consistency. Until platforms and publishers build structured measurement into their systems and actively support its use, marketers will continue to operate in silos. That’s how we stop guessing and start measuring what really matters.
Dig deeper: How AI makes marketing data more accessible and actionable
The post The real reason marketing measurement keeps failing appeared first on MarTech.