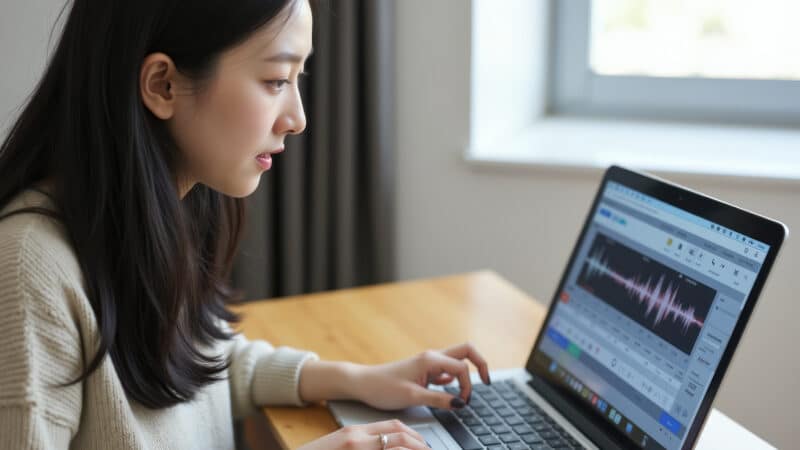
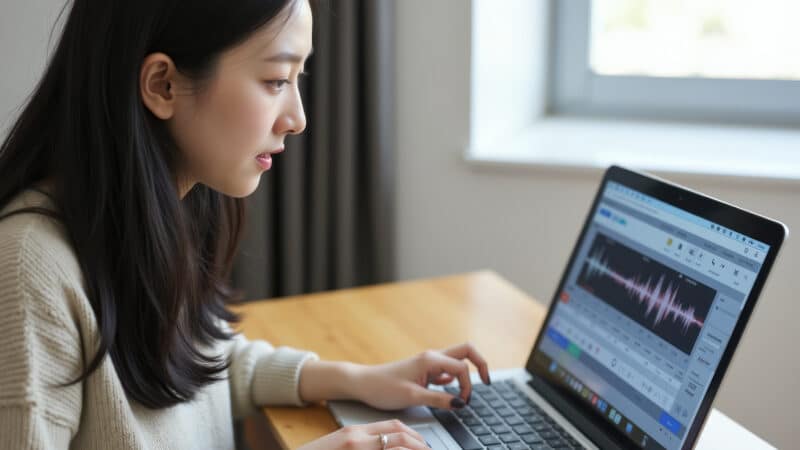
Generative AI (GenAI) has undeniably transformed the marketing function, from automated customer interactions to content creation. But while everyone has been focused on chatbots and creating new blog posts, a quiet revolution has been brewing in Digital Asset Management (DAM). It began with addressing long-standing challenges related to asset findability and reuse but today we are seeing a number of exciting new, high-value use cases that will take us well beyond asset tagging and unlock the true creative potential of your DAM solution.
Asset tagging and retrieval
One of the core tenants of DAM is asset reuse. Why invest time, resource and cost in reproducing an asset that already exists? And yet, for decades, this has remained an elusive and near-impossible goal to achieve. The reason for this is simple: images, video, audio and other rich media assets aren’t self-describing. Unlike text-based objects which can be readily, if not always precisely, searched for, digital assets depend on metadata for retrieval.
Up until now, most meaningful metadata had to be created by humans who would look at an asset and then manually enter the data into prescribed fields, ideally applying the organization’s standard taxonomy and ontology. Ignoring the fact that it is very difficult for one person, not to mention a team, to consistently, accurately and repetitively enter this type of information, most organizations are forced to make trade offs regarding the completeness of metadata entry.
Either they require their creative resources to enter metadata as assets are ingested into a DAM solution — an activity that is almost uniformly resented and often poorly executed — or they employ a librarian or team of librarians to properly attribute assets after they have been ingested into the DAM solution. Due to either user reluctance or cost, most organizations find that it is still very difficult to create sufficient metadata to enable pin-point asset retrieval and to effectively reuse assets.
GenAI solves this problem in two very meaningful ways. First, with GenAI organizations are no longer dependent on humans to properly “tag” or apply metadata to assets. Computer Vision is a particular aspect of artificial intelligence (AI) that enables computers to interpret images, video and other rich media assets.
Utilizing Computer Vision, and particularly Vision-Language Models (VLMs), we can now automatically generate text to describe images and videos. We can also easily convert audio – either audio files or audio tracks for video – into text. As a result, we have a virtually limitless, inexhaustible and inexpensive resource to tag digital assets. These models can be augmented or fine-tuned to provide specific metadata that is unique to your organization or intellectual property – think, for example, about color codes, product IDs or character versions. And, they can be constrained by your organization’s unique taxonomy and ontology.
Further, GenAI can also be tremendously effective for asset retrieval, enabling users to employ natural language to quickly narrow search result sets for highly accurate and efficient asset retrieval.
The result: we can now solve the asset reuse issue ensuring that DAM users can quickly, easily and comprehensively find existing assets.
Beyond tagging: Streamlining asset creation
That’s a pretty extensive overview of how GenAI can address asset findability and reuse. And, as you’ll find, many DAM platforms have begun to incorporate GenAI-powered functionality to intelligently tag assets and enable natural-language searches. But what we’re beginning to see is a whole new set of use cases — beyond tagging and retrieval — that will streamline and accelerate new asset creation and the asset review process.
Asset ideation
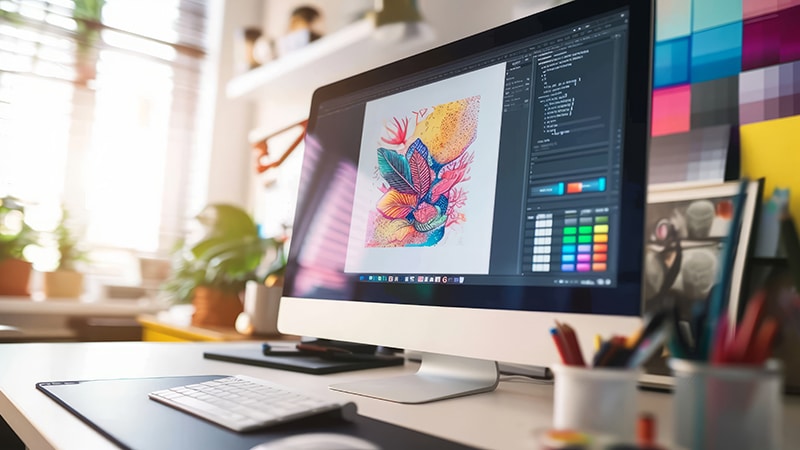
One of the more powerful use cases we are now seeing is asset ideation. With asset ideation, creatives can upload a set of sample assets or intellectual property and then — using a simple, natural language paradigm — provide a set of parameters for new asset ideation. This information is then fed to a Computer Vision model that can rapidly generate a broad array of asset concepts. Then, again using a chat-like interface, users can further refine their results, quickly and easily ideating to identify concepts that work.
By the way, we are emphasizing the word “concepts” here and that GenAI is ideal for ideation, not asset creation. What we have found is that, while Computer Vision models can quickly create any number of new visual assets, most consumers can readily identify assets that are AI-generated and they lack the authenticity of real photos and images.
So the point is to use GenAI for what it is good for: quickly generating an array of concepts to help creative users to conceptualize news assets for a campaign, photo shoot, etc., and then leverage your creative team to produce your final assets. GenAI is not about eliminating the need for creative resources, it’s about providing them with tools to be more effective and efficient.
Asset localization
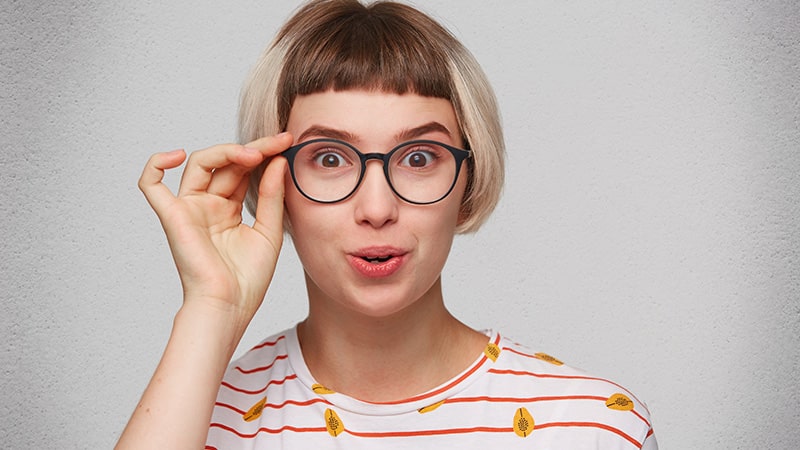
We tend to think about asset localization simply as translation. However, it is much more than this. For global companies, visual assets often need to be localized to align with regional preferences, cultural nuances and even the functional needs of certain segments or geographies. For text, yes, this may involve translation to the local language, but it may also involve localizing currencies and units of measurement, for example. For images and video, you may need to adjust color schemas or incorporate local attire and settings into assets.
GenAI can assist with asset localization in two distinct ways. First and foremost, it can apply localization policies and guidelines to existing assets and flag issues, or it can even identify countries, regions or even specific demographics in which an asset should or should not be used – additional information that can be added to metadata to further enrich the asset. Second, similar to the use case above, GenAI can also be used to create localized concepts and help users to ideate new versions of assets that reflect your policies and guidelines for localization.
Brand compliance
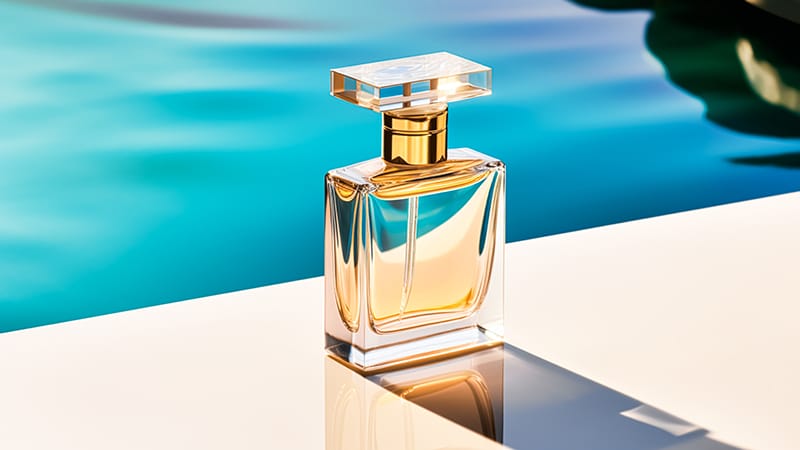
Another valuable use case for GenAI that can also streamline the creative review and approval process is assessing assets for brand compliance. In this use case, as new assets are created and uploaded to the DAM solution, a GenAI model can be used to apply brand policies and guidelines and assess whether or not the asset is in full compliance. In the event that the asset is non-compliant, the model can identify the reasons for non-compliance and even make recommendations as to how to mitigate these issues.
The key thing here is that, as assets are subsequently routed for review and approval, approvers can be assured that the asset is fully brand compliant saving valuable time in review and approval.
Intellectual Property
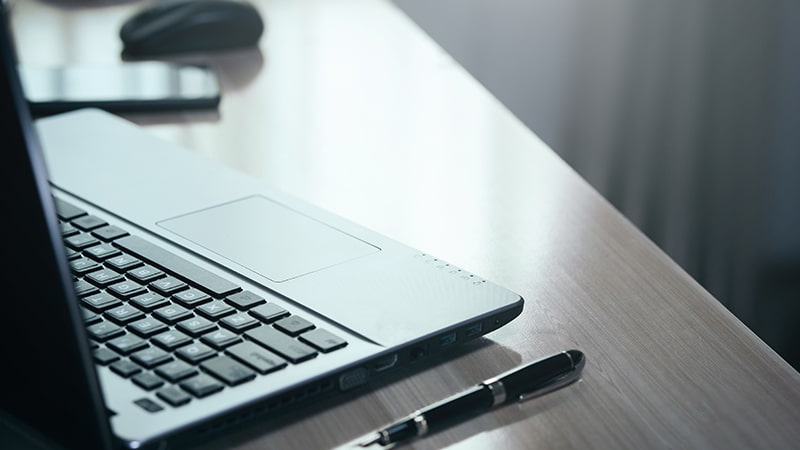
For organizations that utilize third-party intellectual property (IP) in their assets and designs, it is mission critical to understand what IP is being utilized in which assets. It is also crucial to understand when the organization does or does have the right to utilize that IP. This is another value function that GenAI can perform, identifying when an asset contains third-party IP and then validating that the organization has a contractual right to use that IP.
Again, this is valuable metadata that can be generated and applied to an asset in a DAM solution. This is also an automated task that can be run iteratively on existing assets or can be invoked as new assets are added to the DAM solution to ensure that IP rights are never compromised.
This isn’t plug and play
As a final thought, and something I will explore further in future articles, GenAI models are only as good as what they have been trained on. In the early days of AI, we thought this meant that we had to train custom AI models to accurately tag assets or to assess brand compliance. More recently, with methods like Retrieval-Augmented Generation (RAG), we are able to leverage publicly available commercial models for all of the above use cases, though some may still require fine-tuning to optimize accuracy and model outputs.
But the critical thing to understand is that to get accurate, meaningful results with GenAI – even for asset tagging – you have to think about your model inputs and fine-tuning, and this really isn’t out-of-the-box DAM functionality. So, while it’s not as simple as turning on a new feature, there is tremendous value for organizations that get this right and GenAI can truly unlock the potential of your DAM solution.
Learn more about enhancing DAM solutions with generative AI in this complimentary white paper from CMSWire and Vertesia.
The post Beyond tagging: High-value GenAI use cases for DAM appeared first on MarTech.