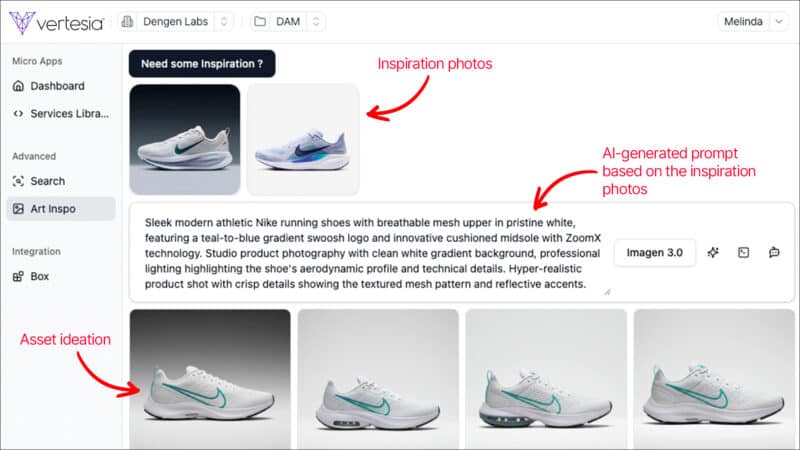
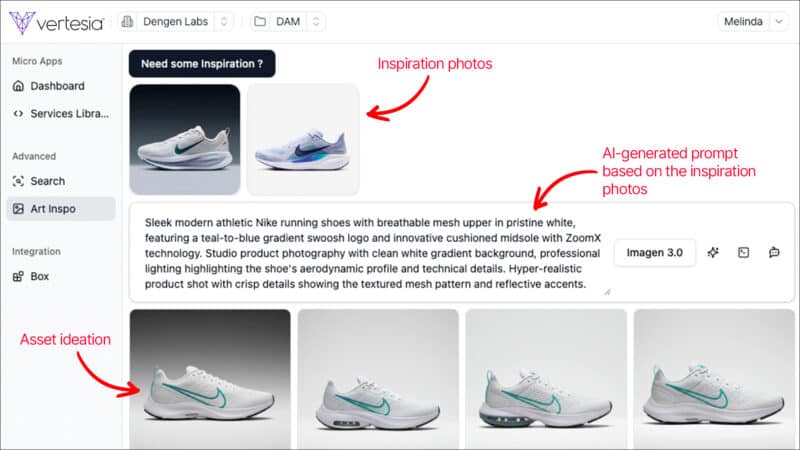
By now, most marketers have at least dabbled with generative AI (GenAI) tools and large language models (LLMs) like ChatGPT, Claude and Gemini. They’ve heard that their competitors are using the technology to practically create entire campaigns with the push of a button. And they’re aware that AI is reshaping marketing and perhaps are both excited and a little worried about what the future will bring.
To date, much of the early conversation about putting AI into production at scale has centered on the need for good prompt engineering — the ability to ask the right questions of this powerful technology. We’ve been told our successful use of the technology hinges on this skill, with the implication we can hire our way out of the problem.
While organizations like big tech companies and AI labs are busy hiring prompt engineers, much of the emphasis on prompt engineering is a holdover from the very early days of GenAI, when familiarity with token length limits, model behavior quirks and methods to avoid hallucinations was unicorn-rare. Today, “prompt engineering” is often just a fancy name for giving an AI model better, more explicit instructions.
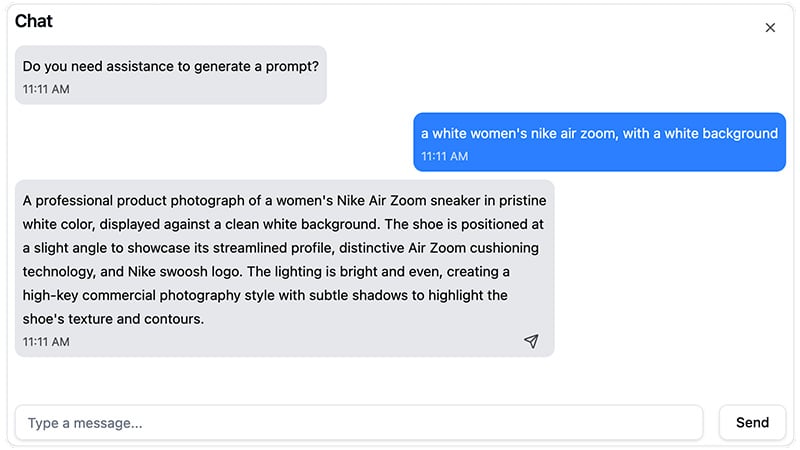
And, as illustrated above, even LLMs are getting pretty good at writing effective prompts.
The truth is, prompt engineering is important—but even the best prompts can’t overcome GenAI’s limitations without RAG.
What is RAG – and why does it matter?
Retrieval-Augmented Generation, or RAG, involves supplying GenAI models with external context — carefully selected data or content — to produce more accurate, relevant and targeted outputs.
One of the biggest challenges with GenAI models is that they will always provide a response, regardless of whether they’ve received the right context or high-quality inputs. Without sufficient data, they frequently produce convincing but inaccurate outputs (hallucinations) or generic, off-brand results that aren’t fit for purpose. RAG directly addresses this problem.
To understand RAG in its simplest form, think of your GenAI model as a fresh-out-of-school new-hire – a resource with enormous potential, skills, and general knowledge, but lacks specific knowledge of your business. RAG acts as the crucial onboarding process—equipping your AI “new-hire” with precise organizational context, brand guidelines, policies and, perhaps most critically, targeted resources and reference information. This “onboarding” transforms your “new-hire’s” basic capabilities into accurate, focused, and brand-aligned outputs, making RAG an essential foundation for enterprises relying on GenAI to deliver business-critical results.
It all begins with data
Just a few years ago, we assumed the only way to get accurate, relevant results from GenAI was to develop custom models. This approach, however, dramatically underestimates the time, complexity, cost and expertise involved. RAG represents a viable and cost-effective alternative.
But first, you need to have an optimized set of data. For traditional text-based marketing use cases, you should curate a collection of existing marketing content that best represents your brand, tone of voice, and how you typically structure different types of collateral and communications. This context will enable a GenAI model to output results that require minimal review and human intervention. However, there are two core challenges here:
- RAG input data needs to be machine-readable. GenAI models perform well with single-page content, but tend to struggle with longer-form, more complex documents, like whitepapers, which typically embed graphics, images, charts and perhaps even tabular data. You need to properly prepare these long-form documents for ingestion by an LLM.
- RAG data must be precisely queryable. A very powerful aspect of RAG is the dynamic “retrieval” of relevant data. However, the effective use of RAG is dependent on being able to retrieve exactly the information you want to feed to your model – nothing more and nothing less.
In short, to get the most out of RAG, you need data that is both well-structured and well-tagged.
One approach to address this need is called “semantic layering” — a precise, structured representation of a document, complete with tables, data extractions for charts and graphics, tabular data and even detailed descriptions of embedded images. XML is a preferred format because it is both easy for GenAI models to understand and provides extensive tagging for document queries. Another advantage of a semantic layer is that it can be reused across multiple use cases and GenAI applications.
As I discussed in my last MarTech article, there are also a number of compelling GenAI use cases related to working with graphics, images, and other digital assets. The requirement for RAG is very much the same here — you need well-tagged inputs with meaningful, structured data that can be readily queried. For example, if you want to use GenAI for asset enrichment utilizing your own taxonomy or ontology and unique metadata (e.g. product IDs, color schemes, etc.), you have to be able to provide the model with the right context to both accurately identify the image content and generate the corresponding metadata.
I would add that while commercial GenAI models can generally identify simple image attributes — like recognizing that an image contains people, a hat or a car — they typically fail to capture nuanced brand aesthetics, cultural references, tone or emotional resonance. Marketers and creative professionals need much more rigorous, detailed asset metadata that aligns with a very specific vision and brand voice.
Simply put, RAG implementation for visual assets demands thoughtful curation and detailed metadata tagging of images and videos, ongoing refinement of visual training data and careful management to ensure outputs remain aligned with evolving creative strategies and brand standards.
Getting GenAI to pay attention
One of the powerful features of RAG is that retrieval (the “R” in RAG) is dynamic, not static. In order to populate your RAG input, you are running a dynamic search based on a given set of parameters to retrieve the most relevant and up-to-date information.
However, an underappreciated issue with most GenAI models is the limited context window. Models have limits (measured in tokens) on the amount of information they can process and understand at one time. Think of it as the model’s short-term memory or working memory. Once you exceed the context window, a model will start to lose track of earlier input, affecting the quality of output. Because of this limitation, marketers cannot simply throw hundreds of documents or thousands of images at a model with RAG and expect it to produce the desired results.
Data preparation enables you to be highly selective with retrieval. Some may argue the superiority of one search technique over another, e.g., graph is better than vector (semantic) search, or vice versa. But I believe that no technique is inherently better than another, and often, the best results come from combining techniques. Regardless, without quality data preparation, no search is going to be terribly accurate.
To be effective with RAG, you need to be able to query a large set of information and select only the necessary data to provide the right context for the model. If you cannot be selective in your retrieval, you risk exceeding the context window (typically 100,000–300,000 tokens) or, even worse, you provide the wrong inputs and context to the model, and the quality of your results suffers. (For reference, one page of text is about 400–500 tokens.)
The best way to deal with GenAI’s limited context windows is to begin with a well-prepared data set and to be very precise, very targeted with your retrieval.
Lighten the load with I=intelligent agents
If all of this sounds like a heavy lift, it certainly can be. A recent survey found that up to 50% of the time spent in building new GenAI apps/agents was attributed to data preparation. However, there is hope. Recently, people have begun to leverage LLMs and GenAI agents to automate data preparation and to create semantic layers.
With a simple prompt, agentic workflows can act autonomously and make use of various tools and techniques to access and retrieve the precise RAG inputs needed to generate the best response for a given task or activity. This approach provides two critical benefits:
- It enables non-technical users, like marketers and creative professionals, to effortlessly leverage generative AI for sophisticated projects, dramatically reducing complexity and learning curves.
- It automates labor-intensive processes, such as data preparation, significantly improving the quality of GenAI outputs and accelerating time-to-value for new GenAI applications.
All of this is why, in my previous article, I made the point that GenAI isn’t “plug and play” – there are deeper complexities and RAG is one. But there are tools and platforms that make this journey quicker and easier, helping to build custom GenAI apps and agents without the inherent costs and complexities.
This isn’t to suggest that RAG will give immediate access to push-button brand campaigns, at least for now. But RAG does present the opportunity to repurpose existing marketing assets in ways that create new value for very little effort — and in a way that retains the brand identity and voice that marketers have worked so hard to establish.
Learn more about agentic RAG with Vertesia.
Written by Chris McLaughlin, Chief Marketing Officer at Vertesia
The post RAG: The most important AI tool marketers have never heard of appeared first on MarTech.