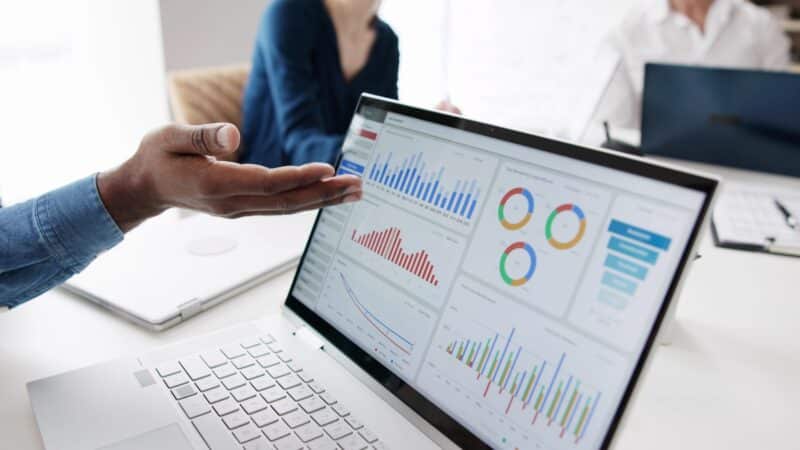
Reliable data underpins every successful martech strategy, giving marketers the clarity needed to make informed decisions about performance and optimization. While the demand for timely, accurate data remains constant, the use of data continues to evolve alongside changing business goals and expectations.
Today, measurement needs to take center stage. Without a clear understanding of effectiveness, there’s no real direction for optimization. But recent shifts — driven by technological advances and tightening privacy regulations — have disrupted the attribution-heavy models marketers have leaned on for over a decade. It’s no longer enough to rely on a single method of measurement.
Instead, marketers must adopt a more flexible, layered approach that reflects both the complexity of the customer journey and the growing limitations of traditional tracking. By combining multi-touch attribution (MTA), marketing mix modeling (MMM) and incrementality testing, it’s possible to build a more complete, accurate picture of what’s truly driving performance.
Each method serves a unique purpose within the broader measurement framework. The key is knowing how and when to use each one.
Multi-touch attribution
Understanding how customers interact and convert through user-level touchpoints remains a crucial part of any marketer’s toolkit. Some level of user-based attribution will always be needed to monitor and optimize daily activity. It supports core bidding and reporting functions across major platforms like Google Ads, Facebook and DV360.
However, evolving regulations and increased scrutiny around user data have created gaps in attribution models. Laws like GDPR in Europe and CCPA in the U.S. have introduced new data sharing and processing limitations, accelerating the shift away from third-party cookies.
As a result, gaining a clear, direct view of user behavior, especially for upper-funnel activity, is becoming increasingly complex. MTA alone can no longer provide the whole picture, making it essential to complement it with alternative measurement approaches.
Dig deeper: How attribution masks what’s actually driving growth
Marketing mix modeling
Even before recent shifts in privacy and tracking, marketing mix modeling had a well-established role in helping marketers understand the broader drivers of sales.
Traditionally, MMM insights were delivered in static reports every 6–12 months, aggregating outputs into broad categories like digital, offline media and TV. That often made it challenging to apply the findings to tactical decisions. But with advances in machine learning, those same capabilities are now available in a more frequent, granular and accessible format, making it easier for brands to onboard and act on insights.
One of MMM’s greatest strengths is its ability to incorporate a wide range of data sources to understand what truly impacts performance. A well-designed model can account for everything from branded search, CTV and upper-funnel Meta campaigns to external factors like inventory, seasonality, pricing and competitor activity.
That lets marketers answer strategic business questions — such as how to allocate budgets across channels, forecast ROI or separate the impact of discounts from marketing efforts. While MTA is best suited for day-to-day optimization, MMM provides a strategic view that supports long-term planning and cross-functional alignment.
Historically, accessing MMM required significant investment in technology and licensing. However, the release of open-source tools like Meta’s Robyn and Google’s Meridian has dramatically lowered the barrier to entry. While these tools still require a blend of data science and marketing expertise, they have democratized advanced measurement — benefiting both seasoned users and brands new to MMM.
Dig deeper: Rethinking media mix modeling for today’s complex consumer journey
Incrementality testing
Despite its strengths, MMM relies heavily on historical data to evaluate performance and forecast future outcomes. However, MMM becomes less effective when launching a new channel or product, where little to no historical data exists,.
That’s where incrementality testing proves its value. Statistically, it remains one of the most robust measurement methods available. Whether through geo experiments or user-level A/B tests, setting up strict control and exposed groups allows marketers to isolate the impact of a specific variable, such as a channel, creative, or pricing change, and measure its actual effect on sales. Like MMM, incrementality testing is unaffected by privacy restrictions, making it a vital part of any brand’s measurement strategy.
A key advantage of incrementality testing is its ability to validate the findings of other measurement methods. For example, a geo experiment can confirm whether shifting budget from affiliates to CTV actually improves ROI. Even when tests don’t yield the expected results, they provide critical input — helping calibrate your MMM model and refine future decision-making.
However, it’s important to remember that incrementality tests are snapshots of a specific moment in time. A substantial uplift from a generic search test last month doesn’t guarantee the same result in the future. To maintain a clear, up-to-date view of marketing effectiveness, brands should run these tests regularly and segment them further wherever possible. Doing so can reveal deeper insights, like which generic keywords drive the most incremental value.
Dig deeper: How smarter measurement can fix marketing’s performance trap
Striking a balance
Choosing the right measurement approach for each objective is essential to making confident, data-driven decisions. All three methodologies — MTA, MMM and incrementality testing — should be central to a brand’s strategy. However, their actual value comes from using them in a coordinated, complementary way, with alignment across teams on what tool is best for each type of decision.
My golden rule for building a measurement strategy is to keep it simple.
- Start with the core business questions you need to answer.
- Match each question to the most appropriate measurement method.
- Establish a process connecting insight generation to decision-making and activation.
When used together — with intention and clarity — these tools can transform your understanding of marketing effectiveness and allow your organization to move with greater precision and impact.
The post What your attribution model isn’t telling you appeared first on MarTech.